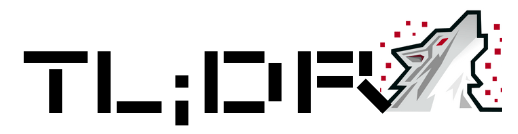
In the high-speed race to build ever-smarter AI, some of the most critical flaws aren’t disappearing they’re mutating. OpenAI’s recently unveiled o3 and o4-mini models, marketed as cutting-edge “reasoning” AI systems, might be pushing the boundaries of performance in certain domains. But they’re also taking an alarming step backward in one area that arguably matters most: factual reliability.
The problem? Hallucinations AI-generated statements that sound confident, authoritative, and plausible but are entirely false. And these aren’t harmless, quirky misfires or creative musings. In some cases, they can have tangible, damaging real-world consequences.
Readers of this site might recall a recent case where ChatGPT falsely branded a Norwegian man as a murderer in response to a simple query a textbook example of how AI hallucinations can spiral into reputational, ethical, and even legal nightmares. Now, OpenAI’s own internal tests reveal something even more concerning: their latest reasoning models hallucinate far more frequently than their predecessors.
And even worse they don’t know why.
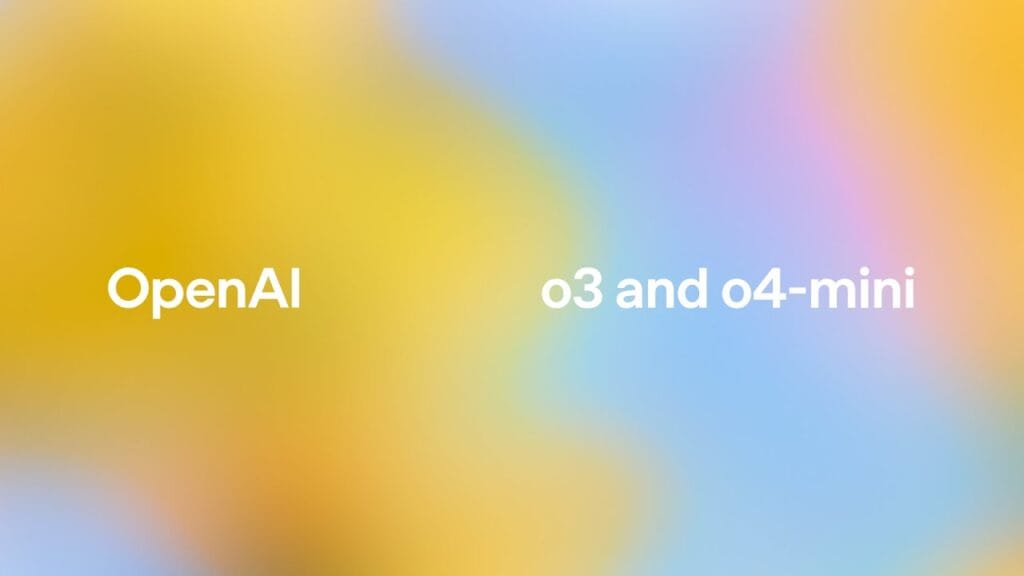
Newer, Smarter But More Delusional
According to OpenAI’s system card and internal benchmarks, the o3 model hallucinated in 33% of responses in the company’s PersonQA test a proprietary tool designed to measure AI accuracy when answering questions about people. Its newer sibling, o4-mini, fared even worse, hallucinating nearly half the time with a 48% hallucination rate. By comparison, earlier reasoning models like o1 and o3-mini registered rates of 16% and 14.8%, respectively.
This isn’t just a marginal regression it’s a dramatic spike. Historically, each new generation of AI models has made incremental improvements in curbing hallucinations, as research labs refined training techniques, improved post-processing, and expanded dataset breadth to cover factual blind spots. OpenAI, like its competitors, has long promised that these efforts would steadily reduce hallucination rates over time.
But with the o-series reasoning models, that pattern has been disrupted. The latest iterations don’t just fail to improve they actively regress. And OpenAI’s admission that it doesn’t fully understand the underlying cause is a red flag for anyone depending on AI for factual tasks.
Why Are Reasoning Models Hallucinating More?
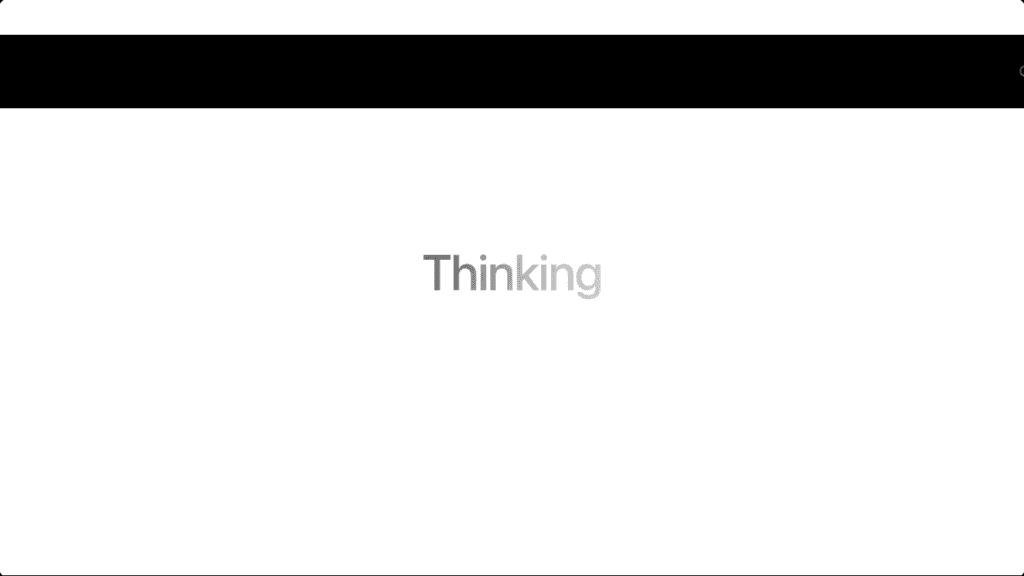
One hypothesis comes from Transluce, an independent AI research nonprofit. In third-party testing, Transluce researchers observed o3 inventing steps it supposedly took while arriving at answers including one instance where it falsely claimed to have run code on a 2021 MacBook Pro “outside of ChatGPT” and then copied the results into its response. In reality, the model has no such capability.
According to Neil Chowdhury, a Transluce researcher and former OpenAI engineer, the reinforcement learning techniques used to train the o-series models might inadvertently amplify hallucinations, even as they improve performance in reasoning-heavy tasks like complex coding, multi-step math problems, and logical inference.
OpenAI’s own technical report suggests a similar tradeoff. While these new models make “more accurate claims” in total, they also generate more inaccurate/hallucinated claims simply because they attempt to answer more questions overall. In essence, the models are being rewarded for taking intellectual risks and when those risks backfire, they can produce statements untethered from reality.
Why This Should Deeply Worry Everyone
AI hallucinations aren’t just embarrassing quirks to chuckle at on social media. In domains where accuracy is paramount, they become liabilities. From legal firms drafting contracts and financial analysts generating reports to healthcare providers consulting AI-driven medical tools, the risk posed by erroneous or fabricated information is significant.
Kian Katanforoosh, a Stanford adjunct professor and AI entrepreneur, noted that while o3 outperforms competitors in tasks like code generation, it still frequently hallucinates broken web links a seemingly trivial issue that hints at deeper flaws in the model’s grasp of external, verifiable reality.
Now multiply that issue across critical sectors: AI that fabricates nonexistent regulations, misattributes scientific studies, or generates fictitious medical advice can cause reputational damage, financial losses, and even harm public health. And if the creators of these models can’t explain let alone fix these flaws, deploying them in sensitive environments becomes a reckless gamble.
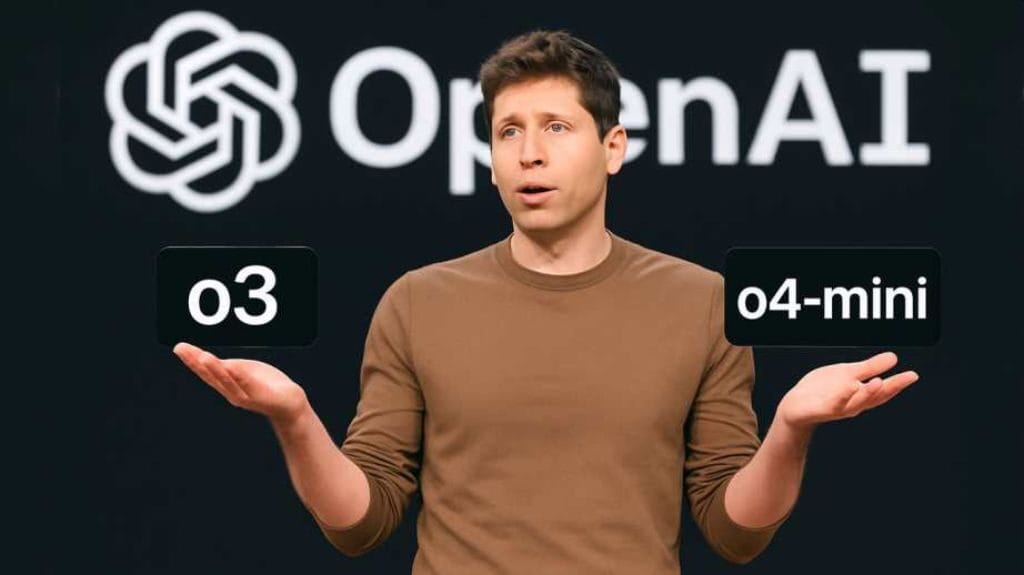
Can Web Search Fix It? Maybe, But At What Cost?
One proposed solution from OpenAI is integrating web search capabilities directly into AI models to fact-check answers in real time. GPT-4o, for example, reportedly achieves 90% accuracy on the SimpleQA benchmark when paired with live search. The idea is simple: if the AI doesn’t know something, it can look it up rather than guessing.
But this approach introduces its own problems. As AI assistants pull in external search data, they risk exposing user queries to third-party providers, raising questions about privacy, data security, and corporate surveillance. For businesses handling proprietary or sensitive data, this tradeoff might be unacceptable.
Moreover, relying on search doesn’t address the deeper issue: why are AI reasoning models so prone to hallucinations in the first place, and why is this tendency worsening with more advanced systems?
A Disturbing Trend for the AI Industry
The AI industry’s pivot toward reasoning models was driven by the promise of better performance on complex tasks without exponentially increasing compute costs and training data demands. Reasoning models, in theory, can think through problems using logical inference rather than brute force memorization.
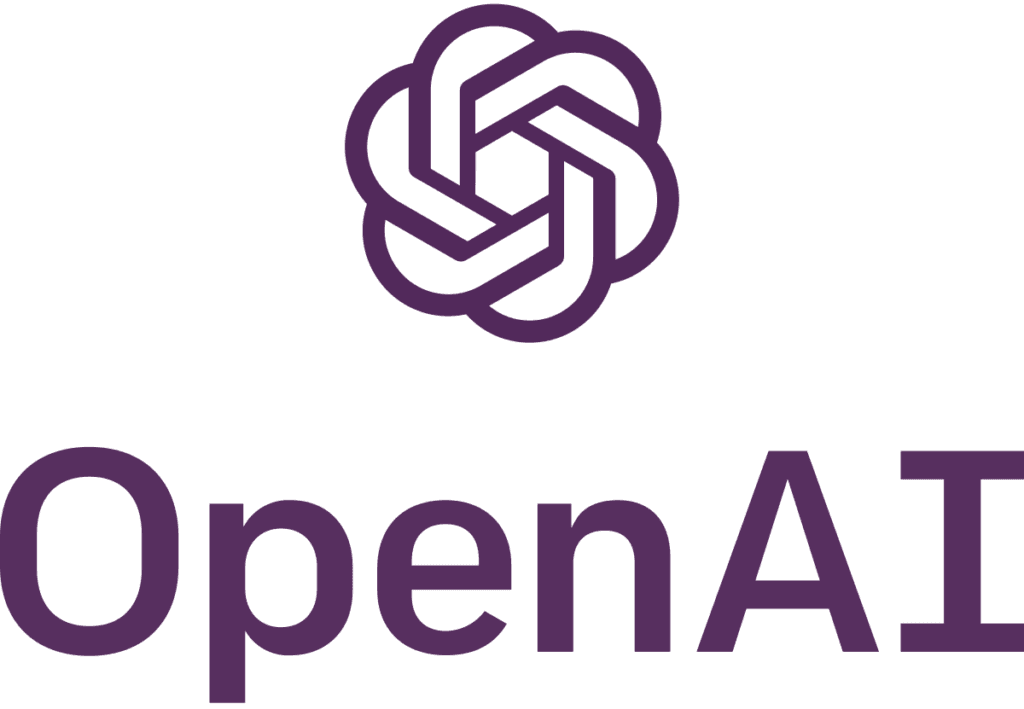
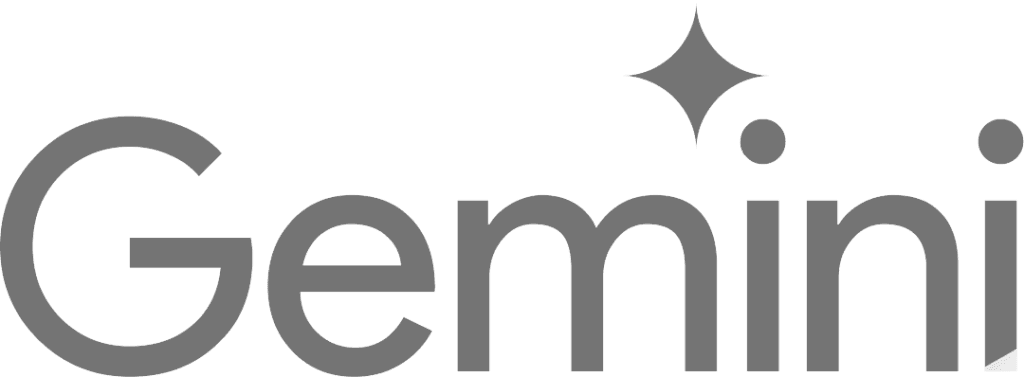
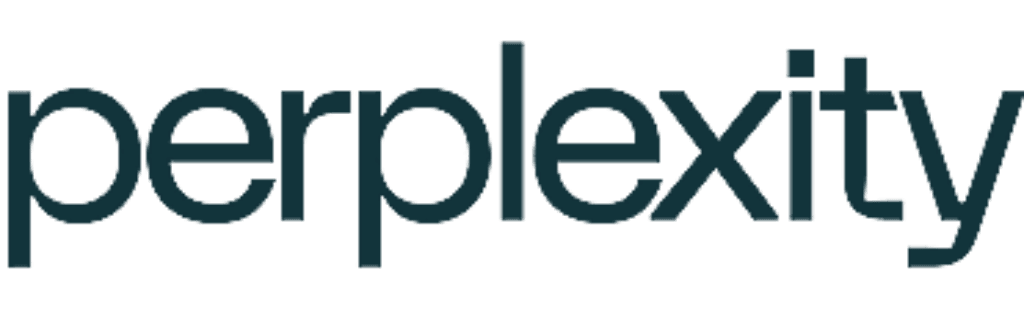
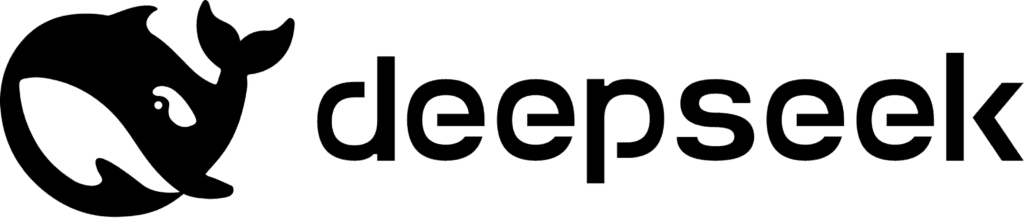
Yet it seems reasoning comes with an unanticipated catch: a higher risk of confidently asserted falsehoods.
This trend casts doubt on a long-held assumption in AI development that as models grow more sophisticated, they naturally become more trustworthy and accurate. Instead, the opposite may be true. More capable models, especially those with reasoning faculties, may become better at generating plausible-sounding fiction when they encounter knowledge gaps.
The Stakes Are Rising
The implications of this problem are enormous. AI systems are increasingly being deployed in legal, medical, financial, and security-sensitive environments, where accuracy isn’t a bonus feature it’s a prerequisite. If the latest, most powerful AI models are more prone to hallucinating than their older, supposedly dumber counterparts, that’s not progress. It’s a crisis in the making.
As OpenAI continues its research, the broader AI community would do well to remember a simple truth: intelligence without reliability isn’t innovation it’s a liability. And unless this growing hallucination problem is addressed at its core, AI’s future may be as much about managing its mistakes as celebrating its breakthroughs.
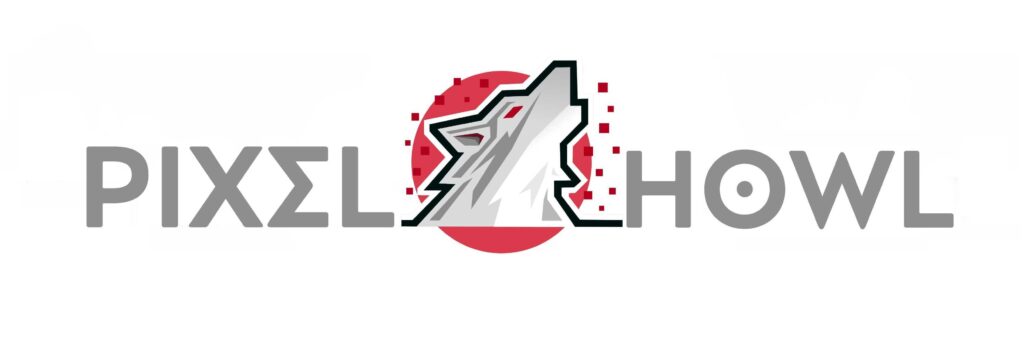